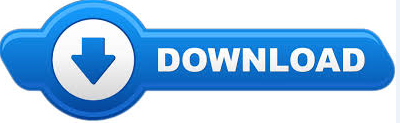
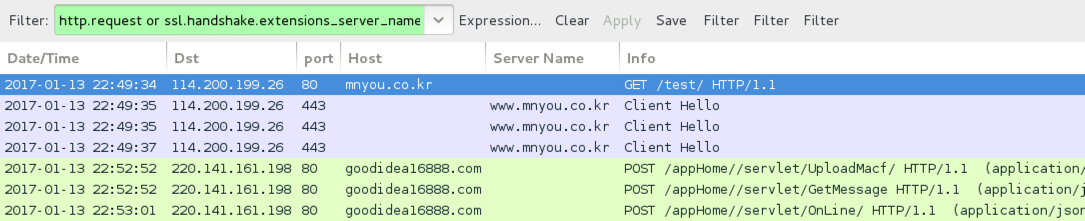
Traffic detection has attracted much attention in recent years, playing an essential role in intrusion detection systems (IDS). In order to overcome this issue, we advise the research community to use our clustering algorithm to get rid of semantically similar apps before evaluating their malware detection mechanism. In our experimentation with Drebin dataset, we found that, after removing the exact duplicate apps from the dataset (? = 0) the malware detection rate (TPR) of API call based ML models is dropped from 0.95 to 0.91 and permission based model is dropped from 0.94 to 0.90. For studying the impact of semantically similar apps in the performance measures, we tested the performance of distinct ML models based on API call and permission features of malware and goodware application with/without semantically similar apps. For this, we propose a novel opcode subsequence based malware clustering algorithm to identify the semantically similar malware and goodware apps. In this paper, we study the impact of semantically similar applications in the performance measures of ML based Android malware detectors. Hence, the performance measures might be seriously affected by these similar kinds of apps. Many researchers unaware about these semantically similar apps and use their features in their ML models for evaluation. Hence, there may exist several semantically similar malware samples in a family/dataset. Malware authors reuse the same program segments found in other applications for performing the similar kind of malicious activities such as information stealing, sending SMS and so on.
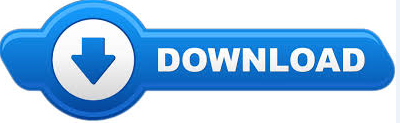